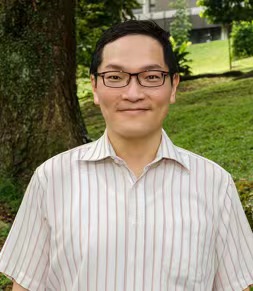
报告人:Chen-Chieh Feng(副教授)
时间:2022.11.25 14:00-15:00
腾讯会议:868-918-66794
密码:见邮件或班级通知
报告人简介
Chen-Chieh Feng is an Associate Professor at the Department of Geography and affiliates with the GIS Unit and the Tropical Environmental Change research group in the same department. He holds a MS from National Taiwan University and a PhD from SUNY-Buffalo, both in Geography. He has published over 50 international refereed journal articles in geographic information science, covering topics such as ontology, spatial cognition, data modelling, and volunteered geographic information, and their applications in environmental modelling, public health, digital humanity, urban transportation, and three-dimensional GIS. He is currently expanding his research interest in spatial ontology to multi-lingual applications through machine learning approaches.
报告简介
Land use is arguably one of the most fundamental sources of information for sustainable urban management. Urban functional zones (UFZs), the spatial units that capture urban socioeconomic functions, reflect nuanced land use patterns in urban settings, and thus the extraction of UFZs has become indispensable in a wide range of urban applications. Recent research on UFZ classification have undoubtedly demonstrated the benefit of integrating multi-source geospatial data, especially those from remote sensing and social sensing means. However, the use of social sensing data, which is inherently user-generated, requires careful handling given the possible heterogeneities and quality issues. In addition, existing research efforts often do not consider urban mobility fully. This study explores the possibility of using a graph-based approach for UFZ identification, where features from high spatial resolution remote sensing images, points of interest, and GPS trajectory data are used. It combines high-level scene information from remote sensing imageries with multisource feature information from physical and social sensing sources, and constructs the graph using mobility information and GPS trajectory data. The proposed approach was tested in Singapore, which achieved an overall accuracy of 84.5%. The approach contributes to heterogeneous data fusion and has the potential to be generalized for other scenarios.